How data products will shape the future of business
One of the fundamental industry trends impacting businesses is that every company is becoming a software and data company. We are in the middle of a once-in-a-generation march towards data and algorithms being forever embedded in internal and customer-facing applications. So what impact will this have on how businesses approach decision making and how they interact with their customers?
The emergence of Data Products
This meta-trend is fuelling the emergence of ‘data products.’ A data product is defined as: “A product where data is explicitly a part of the value delivered to a customer. The underlying data is a point of strategic differentiation enabling a company’s competitive advantage”
The value is delivered in form of personalisation or convenience for end customers or unique insights for business decision making. The differentiation comes in form of a unique data assets, algorithms, or a business model. This results in a competitive advantage for a business in form of customer retention, increased profitability, or creation of competitive moats.
We are surrounded by such products in our daily lives for example – Google Maps, Spotify, QQ Music or JOOX Music, which all offer personalised playlists, experimentation platforms or internal dashboards to us, the end users.
An increasing array of Data Products
Today, wide variety of data products exist to cater to multiple use cases and internal and external audiences. These data products support the entire value chain from managing and structuring raw data to automated platforms for experimentation. The solutions are built and maintained by evolving job families of data engineers, machine learning (ML) engineers, and data scientists. Depending upon the use case, many of these data products are designed to be intuitive enough to be used by audiences without any data expertise.
A booming business landscape
Sensing the growing business demand for data products, the industry has seen a lot of action with the launch of new companies and products, increased venture capital funding and M&A activities. Some of the key areas of focus are data infrastructure, analytics platforms for Business Intelligence (BI) and data visualisation, ML and Artificial Intelligence (AI) platforms such as Google Cloud AI, and enterprise applications. A snapshot view of the complex ecosystem can be found here.
Source: Google ML Academy Webinar
Given the scale of business opportunity, companies are increasingly competing for talent, and inevitably for data scientists and ML engineers. In many areas, demand is outstripping supply, resulting in wage inflation and scarce talent in the market.
Given the lucrative job market and an opportunity to learn these in-demand skills, there has been a significant increase in enrolment for courses related to data and ML skills across platforms such as Coursera.
Based on a survey of 85 Fortune 1000 firms by NewVantage Partners, less than 40% of the organisations surveyed agree that they are managing data as a business asset and create a data-driven organisation. Around 90% of predictive models built in labs never make it to the production environment.
Source : Based on Survey of 85 Fortune 1000 firms by NewVantage Big Data and AI Executive Survey 2021
Given the big difference in the intent and the actual outcomes despite investments in data related infrastructure and talent, business stakeholders are asking the data and technology counterparts the right questions:
What can businesses do?
The starting point for organisations starting out in data products is to develop a nuanced understanding of the value chain of products. This often presents inherent challenges starting with an ill-defined business problem, but clearly defined success KPIs and benchmarks.
As an illustrative example consider the case of a hypothetical grocery delivery app where the business has identified an opportunity to improve customer experience via a personalised pre-populated grocery shopping list on the app. In order to be able to capitalise on this, the insight, business, data science, and technology teams need to be able to come together and answer the below questions even before they start building the solution:
In absence of this rigorous thinking behind answering the above questions, it will be very difficult to get the right solution which works for the end-customers and the business.
As depicted in the example below, businesses need to evaluate every data product with a multidimensional lens.
What does this mean for business and talent?
As the trend of using data and ML to enable business decision making and improve the customer experience unfolds, there are some key implications for professionals in this area as well as businesses investing in setting-up data and ML teams:
- The value of data science will increasingly be realised through data products.
- The business, technology and data science teams will need to work hand-in-hand to build data products which work for the business as well as end customers.
- Professionals investing in product management skills will reap rich rewards in future as data/platform/machine learning product managers.
- With ML democratisation, the value proposition will shift from sheer algorithmic knowledge to domain skills and business outcomes.
TOPICS
The latest insights from our experts around the world
The factors underpinning AI’s success
Learning from the leaders: lessons in best practice from Europe’s winning hypermarkets
dunnhumby’s Global Partner Summit: five things we learned about personalisation and loyalty
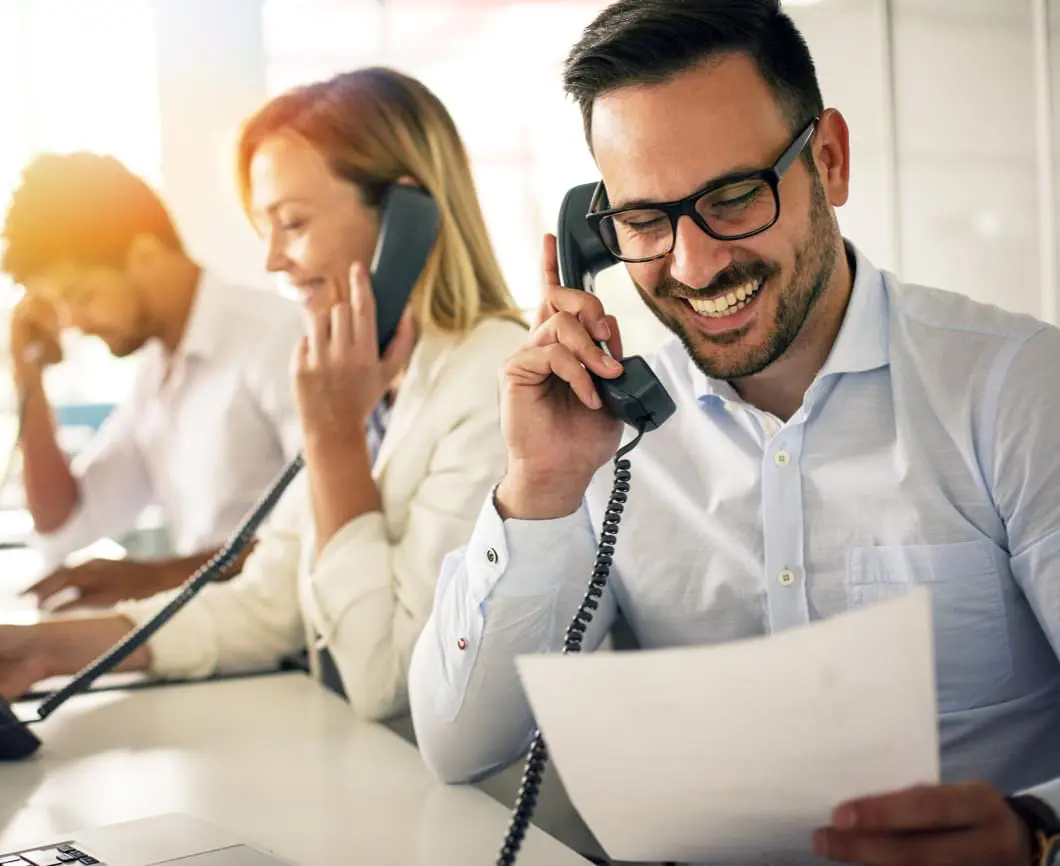