How do grocery retailers deliver the right level of personalisation for customers: Part Two
From the shows we watch to the products we buy, and even the way that we look after our health, personalisation is starting to become commonplace in just about every aspect of our daily lives. Whether we’re being recommended a film based on what we’ve watched before, or having car dashboards built around the way that we drive1, organisations everywhere are tailoring their services around our specific needs.
In grocery retail, the situation is usually a little more complex. Grocery stores operate at a level of frequency and volume that’s unheard of elsewhere – dealing with tens of thousands of items, and millions of individual customers. As a result, the level of personalisation that might work for a clothing store or a bookseller typically isn’t enough to meet the demands of a grocery business.
That’s particularly true when it comes to the concept of predictive personalisation. Here, grocery retailers can use data science to make insightful predictions about which items each customer will need and buy in the future, and issue timely, tailored offers and promotions on those goods. That’s valuable, because it gives customers what they need when they need it, and helps retailers drive sales.
Personalisation at this kind of scale is complex, and isn’t something that the majority of “traditional” CRM and MarTech systems can deliver. At the same time, it’s not uncommon for grocery retailers to invest in those platforms believing that they can. That’s a problem – particularly when a retailer has already gone to a lot of time and expense integrating a system, only to find out that it doesn’t do everything they need it to.
So how do grocery retailers make sure that they’re delivering the right level of personalisation to shoppers? And what do they need to make it a success?
Meeting the needs of millions in real-time
To deliver predictive personalisation at scale, you need three things: rich, long-loop customer data, the right analytical techniques to draw insights from it, and a personalisation system that can simultaneously process and act on those insights.
The data itself is perhaps the most readily available of the three. Most grocery retailers today are already in possession of everything they need, equipped as they are with online and offline purchasing records as well as corresponding loyalty data. Turning that raw information into insights then becomes a case of applying the right data science.
There are many different models that can be used to drive personalisation from customer data, but two in particular are highly effective. The first is what we call “product affinities”, which are something of an inversion of typical categorisation techniques.
In a normal grocery store, products are ordered in a way that makes most sense for a customer when browsing the shelves. Peanut butter, jam, honey, and marmalade will all sit together, for instance. Product affinities disregard that, and instead focus on which items go together in a customer’s basket by using longitudinal behaviour: honey and bread, for instance, or spirits and mixers.
These affinities vary for every retailer in every market, but at their core they help us understand different customer need states, and the way that people shop. That in turn helps us make smart predictions about what they might want next. A customer who frequently buys breakfast pastries might also need butter, coffee, and orange juice, for example.
The second technique that tends to get used here is what can best be described as “people like me” clusters. These clusters group together customers who buy similar products, and provide a weighting against other items. If you and two other people buy 30 similar items, there’s a high chance that you might be interested in something else they’ve bought as well, after all.
Creating a segment of one
Underpinning these models are things like category scores. These provide an overview of how engaged a customer is with a certain category, and are calculated through a deep analysis of an individual’s activity in a specific category to determine their engagement and individual purchase cycle. By comparing their purchase frequency against the average for all shoppers, category scores help retailers understand whether a customer over- or under-indexes in a specific area.
In combination, and with many other machine learning techniques applied, all of this helps to create a “segment of one” for each customer – a comprehensive map of their past behaviours and likely future needs. That map allows us to create propensity scores that tell us how likely someone is to buy a specific item within a given timeframe. This goes all the way down to individual SKU level.
As you can imagine, the scale that we’re talking about here is absolutely massive. dunnhumby’s own science engine generates around 15,000 unique data points for every shopper, and refreshes those daily based on new actions and behaviours. It’s that level of granularity that allows us to create recommendations and personalised offers that are tailored so specifically to individual shoppers.
A great example of this in practice is via our Onsite Sponsored Ads solution. Using the kind of analysis described above, we can predict with some degree of certainty when a customer is most likely to be shopping for a specific item. The nature of Online Sponsored Ads means that brands can bid to have an advert for that product shown to that shopper at just the right time, and in a way that’s entirely natural within the layout of the retailer’s website.
The customer gets what they need when they want it, the brand gets to maximise the effectiveness of their advertising budget, and the retailer makes a sale while also generating media revenues. Everyone wins.
Being pragmatic about predictive personalisation
Scale is one of the biggest challenges facing retailers around predictive personalisation, and not just in terms of the data. As mentioned above, many retailers have started to put their own personalisation foundations in place in the form of CRM systems, despite the fact that these platforms can’t always deliver in the way that they really want them to.
The question then, of course, is whether grocery retailers should take the data they have and attempt to build out their own capabilities in order to process, analyse, and action that information themselves.
I think the best answer here is to think about predictive personalisation in the way that we thought about cloud computing around five or six years ago. While cloud was initially something that retailers were sceptical of, preferring the familiarity of their own hardware and infrastructure, the power, performance and cost-effectiveness the cloud providers bought to the market became impossible to ignore.
Just like cloud, there’ll be a point in the near future where predictive personalisation will be an absolute necessity for any grocery business. And again, just like cloud, there’s little need for grocery retailers to spend the time and money building and maintaining their own systems when highly advanced alternatives already exist.
For the vast majority of grocery retailers, the route to predictive personalisation already exists, with their rich base of customer data serving as the perfect starting point. All that’s needed to start that journey is the ambition to take that first step.
1The Next Level User Experience of Tesla’s Car Dashboard – Adobe Blog
RELATED PRODUCTS
Truly understand your Customers and unlock your Customer First transformation with Strategy Development, Research & Insights and Organisation Engagement
Build a differentiated Customer strategyHelping brands get the most from retail media
Audience Targeting solutionsThe latest insights from our experts around the world
In-house vs. outsource: what’s the right way to run your retail media business?
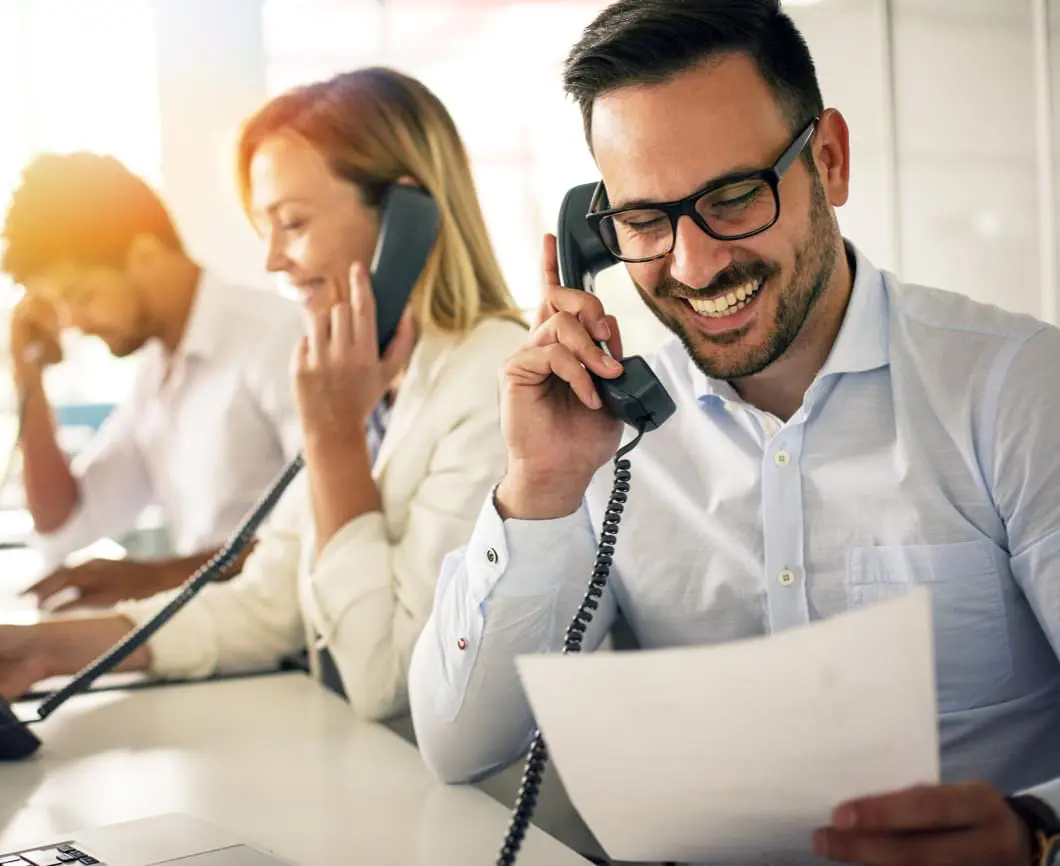